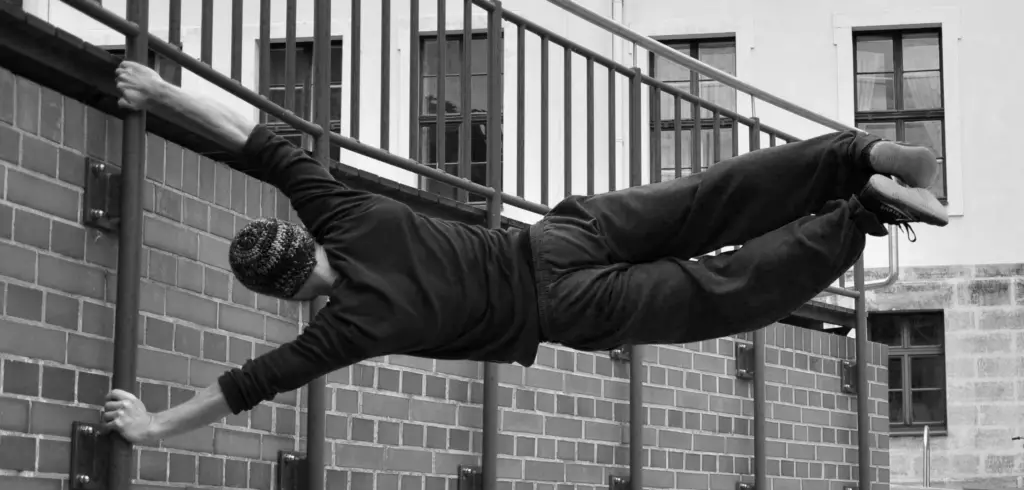
I’m familiar with datasets that—somehow—exhibit normality, meaning they follow a typical bell-shaped curve. With such data, you can build robust statistical models that help make sense of this rollercoaster world. However, when the “rollercoaster” is reflected in your project’s plot, it means your data lacks normality in both senses. Let’s understand why.
The acronym ANOVA stands for Analysis of Variance. In short, it measures the difference between two or more groups. This means the first factor consists of a base group and a group affected by an input (such as a method, treatment, or intervention). However, you also need a second factor that consists of two types of categories:
• Independent category: A category that shares a characteristic (e.g., gender, education type, ethnicity, etc.).
• Dependent category: The result metric that reflects the effect of the input in each group of the first factor.
Learning these concepts can feel nerve-racking at first, but once you master them, you’ve already walked half the path. The next milestone is understanding when to apply this method. Here are the essential conditions your dataset should meet:
1. Consistency – Each group should have the same sample size.
2. Normality – The dataset should follow a Gaussian (normal) distribution.
3. Same Unit of Measurement – The dependent variable should use the same unit across all groups.
With these conditions met, you have a robust methodology to validate whether your hypothesis leads to a significant difference or if it should be rejected. But first things first—check your dataset, visualize it, and if you can structure it properly without major outliers while forming the expected bell curve, then you’re ready to apply ANOVA.